CAN A STUDENT FROM ANOTHER DISCIPLINE STUDY MACHINE LEARNING? | ARTICLE
- Rui Mao
- Apr 30, 2018
- 3 min read
Updated: Nov 11, 2023
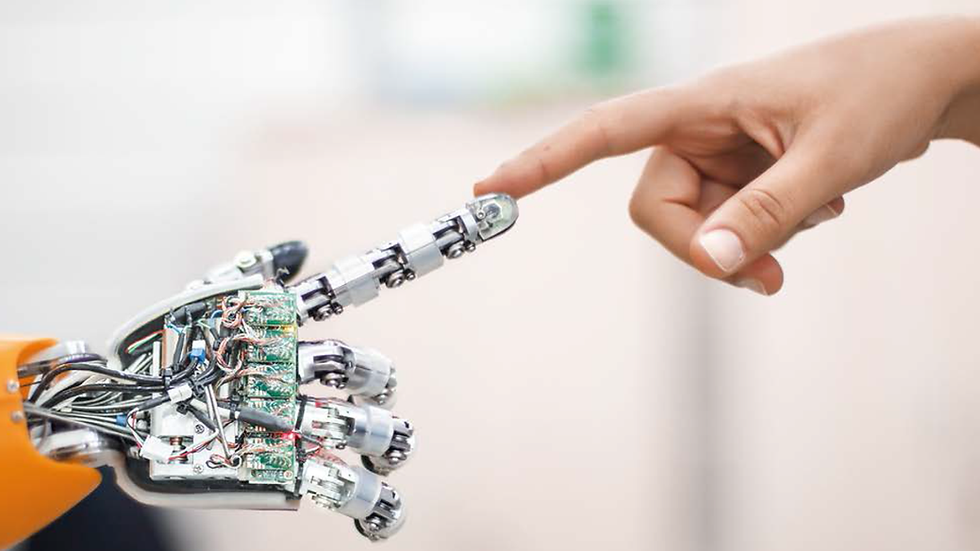
A blanket media coverage of Machine Learning has attracted a large number of Computing Science students to study in this discipline. Challenging, exciting, and promising, all of these characters could be the reason for them to engage in the AI relevant jobs in their future careers. However, if students from other disciplines would like to throw themselves into the AI industries, are they comfortable with the training and the future career challenges? My personal advice is positive. I would like to share my thinking based on my own experience.
I graduated from BSc in Business Administration and MSc in Business Analytics. My undergraduate and master education provides me a good understanding in business and management domain. However, the power of Machine Learning in data mining brings me to Computing Science school to study my Ph.D., as I believe most of business decision makings in the future are data driven. Before the Ph.D was launched, I was worried about the heavy coding work in CS. Although I used R for statistics, I never systematically studied a programming language before. I thought coding may be the barrier, separating CS and non-CS students in ML domain. But this is not true.
The most popular programming language in ML is Python. There are loads of tools written in Python, which helps programmers easily implement their ML algorithms. Python stands out as a high-level programming language, closely resembling human language in its structure and syntax. Gaining a fundamental grasp of Python shouldn't require more than a month, assuming a commitment of two hours per day. Subsequently, you can delve into the basics of machine learning (ML).
Once the first ML algorithm is launched in your practice, you will feel surprised that the machine can be so smart in solving practical problems nowadays. It can mine so much useful information that people can hardly discover from data. For example, market segmentation is critical in business decision. Traditional practices may segment the markets from the perspective of gender, districts, age groups or personal preference, etc. E.g., a newly established fashion design company in China may target to female customer between 20 and 28 who read Vogue. However, such customer profile is illustrated roughly, although they have concerned about their reading habits in their questionnaire survey. With ML, the algorithm, e.g., Decision Tree, may tell the marketing manager that their female customers are English teachers if they rarely cook, or middle class if they do the gym more than twice per week. Such results are given based on analyzing large volume of data (features), so that the machine can capture more interesting business logic.
The given example may recommend a marketing manager to set up their stores nearby the CBD, providing high-price products. English teachers in training institution belong to high income bracket in China, so they would like to pay more in fashionable dresses. They do not cook as they are busy with their works or socializing. Shopping is probably a way of relief for them. Costly dresses may be a burden for middle income young females. But, they may care more about their images, if they go to the gym frequently. They would like to invest more on clothing. Opening stores besides CBD is for the reason that the educational institutions and the best gyms are normally located in this zone.
The power of ML will finally benefit to every industry. In this process, AI enterprises need computing scientists to build the basic facilities, such as algorithms and toolkits. Finding jobs in these firms requires heavy knowledge in CS. However, traditional firms, e.g., in retail, finance and manufacturing industries also need ML technology talented persons. Students or employees from other disciplines can better bring AI into industries, if they would like to study ML. Because they know both the technologies and application scenarios. Personally, this is similar to the role of doctors. We need professional pharmaceutical research scientists to develop new medicines. We also need General Practitioner to give the specific diagnoses for using the medicines, according to the situations of their patients. ML is like a new kind of vitamins. Everyone can learn to use it, as every industry will finally rely on it to strengthen their business and decisions.
Comments